LIMITED TIME OFFER
Replace all of these

with a single tool for just $1 per month for your entire team
UNLIMITED USERS
UNLIMITED PROJECTS
UNLIMITED CHATS
UNLIMITED DOCS
UNLIMITED STORAGE
AND MORE..
Unlocking the Power of Predictive Project Analytics
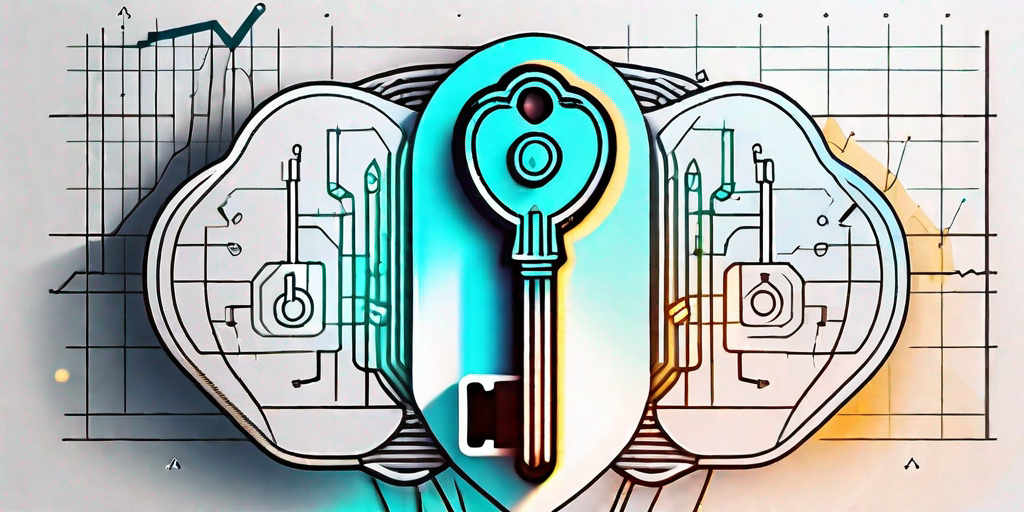
Project managers are constantly seeking new ways to optimize project performance and ensure successful outcomes. One of the most promising tools in their arsenal is predictive project analytics. By harnessing the power of data and advanced statistical models, predictive project analytics allows project managers to make data-driven decisions
, anticipate risks, and identify opportunities for improvement. In this article, we will explore the concept of predictive project analytics, delve into the science behind it, highlight its benefits, discuss implementation strategies, and explore future trends in this exciting field.

Understanding Predictive Project Analytics
Predictive project analytics is a discipline that leverages historical project data, statistical modeling, and machine learning algorithms to forecast project outcomes and facilitate informed decision-making. By analyzing patterns and trends in past project data, predictive project analytics helps identify potential risks, optimize resource allocation, and estimate project timelines and costs. It goes beyond traditional project management approaches, offering insights into the future performance of projects based on historical patterns.
At its core, predictive project analytics is about using data to make informed projections about project outcomes, enabling organizations to proactively address challenges and capitalize on opportunities.
One of the key benefits of predictive project analytics is its ability to provide a holistic view of project performance. By analyzing historical data, project managers can gain valuable insights into the factors that contribute to project success or failure. This knowledge can then be used to develop strategies and make informed decisions that improve project outcomes.
Moreover, predictive project analytics can help project managers optimize resource allocation. By analyzing historical data on resource utilization and project outcomes, project managers can identify patterns and trends that indicate the most effective allocation of resources. This can lead to improved efficiency, reduced costs, and better overall project performance.
Defining Predictive Project Analytics
Predictive project analytics can be defined as the practice of using historical project data, statistical modeling, and machine learning algorithms to predict project outcomes and optimize project performance. It goes beyond descriptive and diagnostic analysis, which focus on understanding what happened and why it happened, to provide a forward-looking perspective.
By leveraging advanced statistical techniques and machine learning algorithms, predictive project analytics can identify patterns and correlations in project data that may not be immediately apparent to project managers. This enables project teams to make more accurate predictions about project outcomes and take proactive measures to ensure project success.
Furthermore, predictive project analytics can help project managers estimate project timelines and costs more accurately. By analyzing historical data on project duration, resource utilization, and cost, project managers can develop models that predict the time and resources required for future projects. This can help organizations plan and budget more effectively, reducing the risk of project delays and cost overruns.
The Role of Predictive Analytics in Project Management
Predictive project analytics plays a crucial role in project management by enabling project managers to make informed decisions, allocate resources effectively, and identify potential risks and bottlenecks. It helps project teams anticipate challenges, adjust project plans, and mitigate risks before they impact project timelines and budgets. By leveraging data and analytics, project managers can make proactive decisions that drive project success.
One of the key advantages of predictive project analytics is its ability to identify potential risks and bottlenecks early in the project lifecycle. By analyzing historical project data and identifying patterns that are indicative of risks, project managers can take proactive measures to mitigate these risks and ensure project success. This can include adjusting project plans, allocating additional resources, or implementing contingency measures.
Moreover, predictive project analytics can help project managers optimize resource allocation by identifying areas where resources are underutilized or overutilized. By analyzing historical data on resource allocation and project outcomes, project managers can identify inefficiencies and make adjustments to ensure optimal resource allocation. This can lead to improved project performance, reduced costs, and increased overall efficiency.
In conclusion, predictive project analytics is a powerful tool that enables project managers to make informed decisions, optimize resource allocation, and identify potential risks and bottlenecks. By leveraging historical project data, statistical modeling, and machine learning algorithms, organizations can gain valuable insights into project performance and make proactive decisions that drive project success.
The Science Behind Predictive Analytics
Predictive analytics is a complex field that combines statistical modeling, machine learning algorithms, and data analysis techniques to make accurate predictions about future events. At its core, predictive analytics relies on historical data and mathematical models to identify patterns, trends, and relationships that can be used to forecast outcomes.
Let’s dive deeper into the fascinating world of predictive analytics and explore how it works and its key components.
How Predictive Analytics Works
Predictive analytics starts with data collection and preprocessing. This involves gathering relevant project data from various sources, such as customer databases, social media platforms, and transaction records. The collected data may include variables such as customer demographics, purchase history, and online behavior.

Once the data is collected, it undergoes a crucial preprocessing step. This step includes cleaning and transforming the data to ensure its quality and consistency. Data cleaning involves removing any outliers, errors, or duplicates that may affect the accuracy of the predictions. Data transformation may involve converting categorical variables into numerical ones or normalizing the data to make it suitable for analysis.
After the data is prepared, statistical models and machine learning algorithms are applied to identify patterns and build predictive models. Statistical models, such as linear regression or time series analysis, use historical data to establish relationships between variables and make predictions based on those relationships. Machine learning algorithms, on the other hand, can automatically learn from the data and adapt their models to make accurate predictions.
Once the predictive models are built, they need to be validated and refined using historical data. This validation process ensures that the models are reliable and can accurately predict future outcomes. The models are tested against known historical data to measure their performance and make necessary adjustments.
Finally, the validated models are used to predict future project outcomes and inform decision-making processes. These predictions can help businesses optimize their operations, identify potential risks, and make data-driven decisions to achieve their goals.
Key Components of Predictive Analytics
Predictive analytics involves several key components that work together to ensure accurate predictions and actionable insights.
Data collection and preprocessing: This component involves gathering relevant data from various sources and preparing it for analysis through cleaning and transformation.
Statistical modeling: Statistical models use historical data to identify patterns and relationships between variables, enabling accurate predictions.
Machine learning algorithms: Machine learning algorithms automatically learn from the data and adapt their models to make accurate predictions without explicit programming.
Model validation: This component ensures that the predictive models are reliable and can accurately predict future outcomes. Validation involves testing the models against known historical data and making necessary adjustments.
Prediction: The final component involves using the validated models to predict future project outcomes and inform decision-making processes.
By leveraging the power of predictive analytics, businesses and organizations can gain valuable insights into their operations, customers, and markets. These insights can help them make informed decisions, optimize processes, and stay ahead of the competition.
Benefits of Predictive Project Analytics
The adoption of predictive project analytics offers numerous benefits for organizations seeking to enhance their project management capabilities. Let’s explore some of the key benefits:
Improving Project Success Rates
Predictive project analytics enables project managers to identify potential risks early on and take proactive measures to mitigate them. By being able to anticipate challenges and adjust project plans accordingly, organizations can increase their chances of project success. It also helps optimize resource allocation, ensuring that the right resources are deployed at the right time, thus improving overall project efficiency and success rates.
Enhancing Decision-Making Processes
With predictive project analytics, project managers have access to valuable insights that empower them to make data-driven decisions. By leveraging historical project data and predictive models, project managers can evaluate different scenarios, assess the impact of potential decisions, and make informed choices. This leads to improved decision-making processes, reduced uncertainty, and better project outcomes.
Implementing Predictive Project Analytics
Implementing predictive project analytics requires careful planning and execution. Here are the key steps to incorporate predictive analytics into project management:

Steps to Incorporate Predictive Analytics
- Evaluate Data Readiness: Assess the availability and quality of project data. Define data requirements and identify any gaps or limitations.
- Data Collection and Preprocessing: Gather relevant project data from various sources and ensure it is clean, consistent, and ready for analysis.
- Statistical Modeling and Algorithm Selection: Identify suitable statistical models and machine learning algorithms that align with project goals and data characteristics.
- Model Training and Validation: Train the predictive models using historical project data, validate their accuracy, and fine-tune them to ensure optimal performance.
- Prediction and Decision-Making: Use the trained models to predict project outcomes, evaluate different scenarios, and inform decision-making processes.
Overcoming Implementation Challenges
Implementing predictive project analytics may come with its fair share of challenges. Some common challenges include data quality and availability, technical infrastructure requirements, and change management. It is important to address these challenges proactively by investing in data governance, ensuring data integrity, building robust technical infrastructure, and educating project stakeholders on the value of predictive project analytics.
Future Trends in Predictive Project Analytics
Predictive project analytics is a rapidly evolving field, and several trends are shaping its future. Let’s explore two exciting trends:
The Impact of AI on Predictive Analytics
Artificial Intelligence (AI) is revolutionizing the field of predictive analytics. AI-powered algorithms can process vast amounts of project data, identify complex patterns, and make accurate predictions in real-time. As AI continues to advance, it will enhance the capabilities of predictive project analytics, enabling more accurate forecasts, improved decision-making, and better project outcomes.
Predictive Analytics in the Era of Big Data
The proliferation of big data has opened up new possibilities for predictive project analytics. With the availability of large and diverse datasets, project managers can gain deeper insights into project dynamics, uncover hidden patterns, and make more accurate predictions. By leveraging big data, predictive project analytics will continue to evolve and offer even greater value to organizations.
Conclusion
Predictive project analytics is a powerful tool that empowers project managers and organizations to unlock the full potential of their projects. By leveraging historical project data, statistical modeling, and machine learning algorithms, predictive project analytics enables informed decision-making, improves project success rates, and enhances overall project management capabilities. The future of predictive project analytics is bright, with AI and big data continuing to drive advancements in this exciting field. Organizations that embrace predictive project analytics stand to gain a competitive edge, enabling them to deliver successful projects and achieve their strategic objectives.