LIMITED TIME OFFER
Replace all of these

with a single tool for just $1 per month for your entire team
UNLIMITED USERS
UNLIMITED PROJECTS
UNLIMITED CHATS
UNLIMITED DOCS
UNLIMITED STORAGE
AND MORE..
Assessing the Effectiveness of Document Tagging
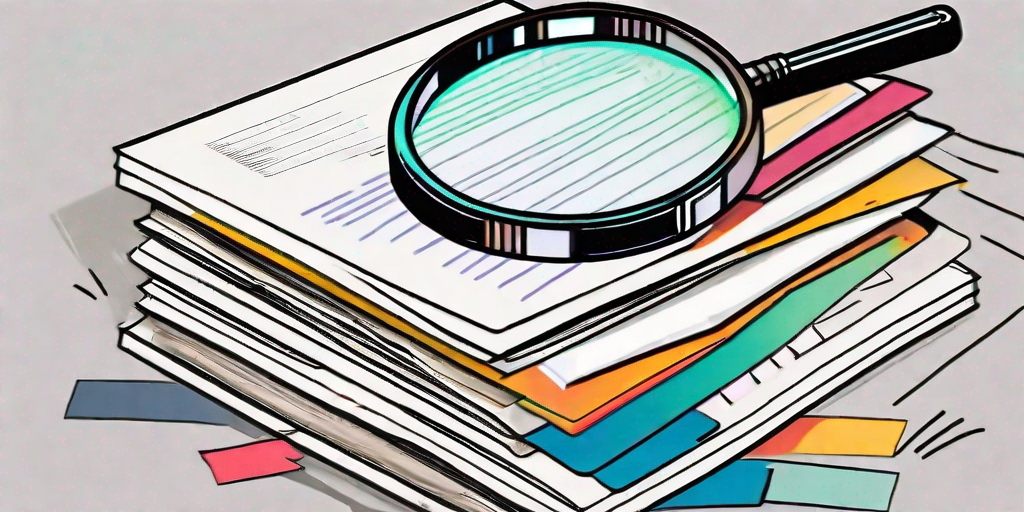
Document tagging plays a vital role in organizing and retrieving information efficiently. Effective document tagging allows users to easily locate and access specific documents based on relevant tags or keywords. However, evaluating the effectiveness of document tagging can be a challenging task. In this article, we will explore the basics of document tagging, methods for assessing tagging effectiveness, challenges in the assessment process, and strategies for improving document tagging effectiveness. We will also discuss future trends in document tagging and its potential impact on information management.
Understanding Document Tagging
Document tagging involves assigning descriptive labels or tags to documents to facilitate easy categorization and retrieval. By associating keywords or metadata with each document, tagging enables users to search for specific information based on relevant tags. It helps in organizing large volumes of documents and enhances information retrieval efficiency. Understanding the fundamentals of document tagging is essential for assessing its effectiveness.
The Basics of Document Tagging
In its simplest form, document tagging involves manually assigning tags to documents. These tags can be descriptive words, phrases, or codes that represent the content or nature of the document. For example, a document related to project management might be tagged with keywords like “project management,” “planning,” “team collaboration,” etc. This allows users to retrieve all relevant documents by searching for any of these tags.
However, document tagging goes beyond just assigning a few keywords. It requires a deep understanding of the document’s content and context. Taggers need to analyze the document thoroughly, extracting key concepts and identifying the most relevant terms to use as tags. This process involves careful consideration and sometimes collaboration with subject matter experts to ensure accurate and effective tagging.
Additionally, document tagging can be automated using machine learning algorithms. These algorithms analyze the content of the document and automatically assign relevant tags based on patterns, keywords, and other factors. Automated tagging can save time and effort, especially when dealing with large document repositories. However, it may not always be as accurate as manual tagging, as it relies on predefined rules and algorithms.
Importance of Document Tagging in Information Retrieval
Efficient information retrieval relies on accurate and comprehensive document tagging. When documents are properly tagged, users can quickly locate and access relevant information, which improves productivity and decision-making. Without effective tagging, finding specific documents becomes time-consuming and frustrating, leading to decreased efficiency and potential information loss within organizations.
Moreover, document tagging allows for advanced search capabilities. Users can combine multiple tags to narrow down their search results and find highly specific information. This flexibility enables users to explore different dimensions of the document repository and uncover hidden connections or patterns that may not be apparent at first glance.
Furthermore, document tagging plays a crucial role in knowledge management systems. By tagging documents with relevant keywords, organizations can create a structured and interconnected knowledge base. This knowledge base becomes a valuable resource for employees, enabling them to tap into the collective expertise and experience of the organization. It promotes knowledge sharing, collaboration, and innovation, ultimately leading to improved organizational performance.
In conclusion, document tagging is a vital process for organizing and retrieving information effectively. Whether done manually or through automated means, accurate tagging enhances information retrieval efficiency and improves decision-making. By understanding the basics of document tagging and its importance in information retrieval, organizations can optimize their document management systems and unlock the full potential of their knowledge assets.
Methods for Assessing Tagging Effectiveness
Assessing the effectiveness of document tagging requires a combination of quantitative and qualitative evaluation techniques. By using these methods, organizations can gain valuable insights into the reliability and usefulness of their tagging systems.
When it comes to quantitative evaluation techniques, there are several key factors to consider. One important aspect is the accuracy of tag assignments. This involves analyzing how well the tags match the content of the documents. By examining the precision and recall of the tags, organizations can determine if the tagging system is correctly identifying and assigning relevant tags.
Consistency in tagging across documents is another crucial factor to evaluate. It is important to ensure that similar documents are tagged consistently, as this helps in organizing and retrieving information effectively. By analyzing the consistency of tags across a dataset, organizations can identify any inconsistencies or discrepancies that may exist.
Furthermore, assessing the search retrieval effectiveness is an essential part of quantitative evaluation. This involves measuring how well the tagging system performs in retrieving relevant documents based on user queries. By analyzing search retrieval metrics such as precision, recall, and F1 score, organizations can gauge the overall effectiveness of their tagging system in facilitating information retrieval.
On the other hand, qualitative evaluation techniques focus on subjective assessments of tagging effectiveness. These methods aim to gather feedback from users to understand their perception of the tagging system’s usability, relevance, and overall experience.
One common qualitative evaluation method is conducting surveys. Surveys allow organizations to collect feedback from a large number of users and gain insights into their satisfaction levels, perceived usefulness of tags, and suggestions for improvement. By analyzing survey responses, organizations can identify patterns and trends in user feedback, helping them make informed decisions about their tagging system.
Another qualitative evaluation technique is conducting interviews. Interviews provide an opportunity to have in-depth conversations with users, allowing organizations to gain a deeper understanding of their experiences and perspectives. Through interviews, organizations can uncover specific pain points, gather detailed feedback, and explore potential areas for improvement.
Usability studies are also valuable qualitative evaluation methods. These studies involve observing users as they interact with the tagging system and collecting data on their behavior, preferences, and challenges. Usability studies can provide valuable insights into user workflows, identify usability issues, and help organizations optimize their tagging system for a better user experience.
Overall, a combination of quantitative and qualitative evaluation techniques is essential for assessing tagging effectiveness. By analyzing measurable criteria and gathering user feedback, organizations can continuously improve their tagging systems, ensuring they are reliable, accurate, and user-friendly.
Challenges in Document Tagging Assessment
Despite its benefits, assessing document tagging effectiveness poses certain challenges that need careful consideration. Being aware of these challenges helps organizations overcome obstacles and make informed decisions about improving their tagging systems.
Inconsistencies in Tagging
Inconsistent tagging can hinder effective information retrieval. Different users may use different terminologies or interpret tags differently, leading to confusion and inaccurate search results. It is essential to establish tagging conventions and guidelines to ensure consistent and standardized tagging practices across documents and users.
One common challenge organizations face is the lack of a centralized tagging system. Without a centralized system, different departments or individuals may develop their own tagging methods, resulting in inconsistencies. For example, one department may use abbreviations or acronyms as tags, while another department prefers full words or phrases. This inconsistency can make it difficult for users to find relevant documents when searching using specific tags.
Another challenge related to inconsistent tagging is the use of synonyms. Different users may use different synonyms to describe the same concept, leading to fragmented search results. For instance, one user may tag a document about “customer satisfaction” as “client happiness,” while another user may tag it as “customer contentment.” This inconsistency in synonyms can create confusion and make it harder for users to locate all relevant documents on a particular topic.
Dealing with Ambiguity in Tags
Tags can sometimes be ambiguous or have multiple meanings, complicating the retrieval process. For example, the tag “Java” can refer to both a programming language and a geographic location. To address this challenge, context-aware tagging techniques and advanced algorithms that consider document context and user preferences can be employed.
Context-aware tagging involves analyzing the content of a document and its surrounding context to determine the most appropriate tags. For instance, if a document contains code snippets and programming-related terms, the tag “Java” is more likely to refer to the programming language rather than a geographic location. By taking into account the document’s context, the tagging system can provide more accurate and relevant search results.
Advanced algorithms can also help mitigate the ambiguity in tags. These algorithms can analyze the frequency and co-occurrence of tags within a document collection to infer their intended meanings. For example, if the tag “Java” frequently appears alongside other programming-related tags like “Python” and “C++,” it is more likely to refer to the programming language. By leveraging statistical analysis and machine learning techniques, the tagging system can better understand the intended meaning behind ambiguous tags.
Furthermore, user preferences can play a role in addressing tag ambiguity. By allowing users to customize their search settings and preferences, the tagging system can prioritize certain meanings or filter out irrelevant results based on individual user preferences. This customization empowers users to tailor the tagging system to their specific needs and enhance the accuracy of search results.
Improving Document Tagging Effectiveness
To enhance document tagging effectiveness, organizations can adopt various strategies and best practices. These practices aim to improve the quality, consistency, and relevance of tags, and incorporate advancements in AI and machine learning.
Best Practices for Document Tagging
Implementing best practices for document tagging ensures that tags are meaningful, accurate, and consistent. Some recommended practices include using descriptive and specific tags, avoiding redundancy, regularly reviewing and updating tags, and training users on effective tagging techniques.
Role of AI and Machine Learning in Tagging
Advancements in AI and machine learning have revolutionized document tagging. Automated tagging systems can analyze document contents, identify relevant keywords, and suggest appropriate tags. Machine learning algorithms can learn from user feedback and continuously improve tagging accuracy and relevance. Integrating AI and machine learning technologies into document tagging workflows can significantly enhance tagging effectiveness.
Future Trends in Document Tagging
As technology continues to evolve, document tagging is expected to undergo further advancements. These advancements will shape the future of information management and enhance the effectiveness of document tagging.
Predicted Developments in Tagging Techniques
Tagging techniques are predicted to become more sophisticated and context-aware. Natural language processing and semantic tagging will enable systems to understand the meaning and context of documents, leading to more accurate and relevant tagging. Incorporating user feedback and preferences will further refine tagging techniques.
Impact of Emerging Technologies on Document Tagging
Emerging technologies such as blockchain, IoT, and augmented reality have the potential to revolutionize document tagging. They can enable seamless integration of document tagging with other processes, enhance metadata management, and provide innovative ways to visualize and interact with tagged information. These technologies will reshape how information is organized, retrieved, and utilized.
In conclusion, assessing the effectiveness of document tagging is crucial for efficient information retrieval. By understanding the basics of document tagging, employing appropriate evaluation techniques, addressing challenges, and implementing best practices, organizations can enhance the effectiveness of their tagging systems. Furthermore, leveraging advancements in AI and machine learning and staying updated with future trends in document tagging will ensure organizations stay at the forefront of information management.